Radiomics is a new and exciting field in cancer research. It uses advanced computer technology to study images from medical scans like CT, MRI, and PET. These images help doctors see what’s happening inside the body. By analyzing these images in a detailed way, radiomics can find patterns that might not be visible to the naked eye. When we combine this information with data about the genes in our bodies (genomics), we can learn even more about how cancer works and how to treat it better.
What is Radiomics?
Radiomics involves looking very closely at medical images to find tiny details. Think of it like a high-tech version of looking through a magnifying glass. These details, called radiomic features, can tell us a lot about a tumor, such as its size, shape, and texture. By collecting and studying these features, scientists can find patterns that help predict how a tumor might behave.
Integrating Radiomics with Genomics
Genomics is the study of all the genes in our bodies. Genes are similar to instructions that tell our bodies how to work. In cancer, some genes might change or mutate, causing the cancer to grow. By combining radiomic data with genomic data, we can get a more complete picture of the cancer.
For example, radiomics might show that a tumor has a rough texture, while genomics might reveal a mutation in a specific gene. Together, this information can help doctors decide on the best treatment plan.
Why is This Important?
Combining radiomics and genomics can lead to better, more personalized treatments for cancer patients. It can help doctors understand which treatments will work best for each patient, based on the unique characteristics of their tumor.
Real-World Examples
- Predicting Treatment Response: Scientists have found that certain radiomic features can predict how well a tumor will respond to treatment. For example, tumors with a lot of irregular edges might not respond well to certain types of chemotherapy. By knowing this in advance, doctors can choose a different treatment that might be more effective.
- Tracking Tumor Changes: Radiomics can help monitor how a tumor changes over time. If a tumor starts to shrink, the radiomic features will change too. This can help doctors see if the treatment is working without needing a biopsy.
Data and Graphs
Here are some examples of how radiomic data can be visualized:
1. Graph Showing Tumor Response to Treatment:
This graph shows how radiomic features can predict a tumor’s response to treatment. The blue line represents tumors with smooth edges, which respond well to treatment. The red line represents tumors with irregular edges, which do not respond as well.
2. Table of Radiomic Features:
Radiomic Feature | Description | Example Tumor Type |
Texture | How smooth or rough the tumor looks | Smooth: Breast cancer |
Shape | The outline of the tumor | Irregular: Lung cancer |
Intensity | How bright or dark the tumor appears | Bright: Liver cancer |
Conclusion
Radiomics is helping us understand cancer in new and powerful ways. By combining it with genomics, we can get a complete picture of what’s happening inside the body. This leads to better, more personalized treatments and gives patients the best chance of beating cancer.
By continuing to develop and refine these technologies, the future of cancer treatment looks promising, with more precise and effective methods on the horizon.n integral part of your overall health, and staying informed ensures that you can address any concerns promptly and effectively.
References
- Lambin, P., et al. (2012). Radiomics: extracting more information from medical images using advanced feature analysis. European Journal of Cancer, 48(4), 441-446.
- Aerts, H. J. W. L., et al. (2014). Decoding tumour phenotype by noninvasive imaging using a quantitative radiomics approach. Nature Communications, 5, 4006. Link
- Gillies, R. J., Kinahan, P. E., & Hricak, H. (2016). Radiomics: Images Are More than Pictures, They Are Data. Radiology, 278(2), 563-577.
- Yip, S. S. F., & Aerts, H. J. W. L. (2016). Applications and limitations of radiomics. Physics in Medicine and Biology, 61(13), R150-R166.
- Parmar, C., et al. (2015). Radiomic machine-learning classifiers for prognostic biomarkers of head and neck cancer. Frontiers in Oncology, 5, 272.
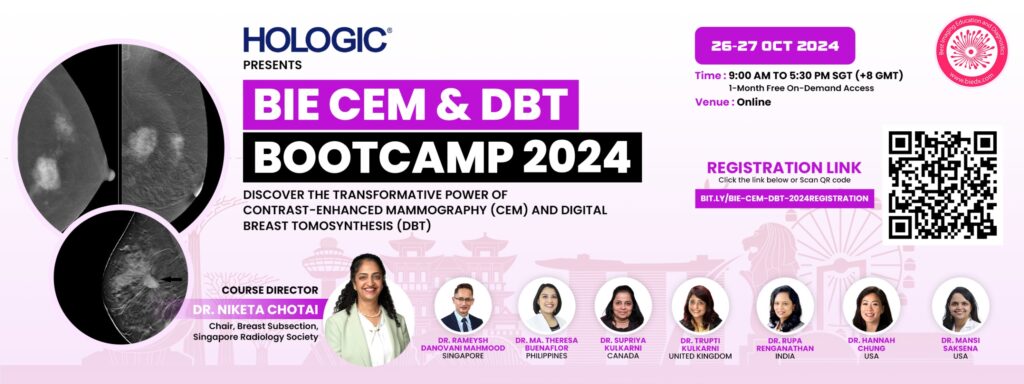